Modeling How Species Speak
Gašper Beguš, PhD '18
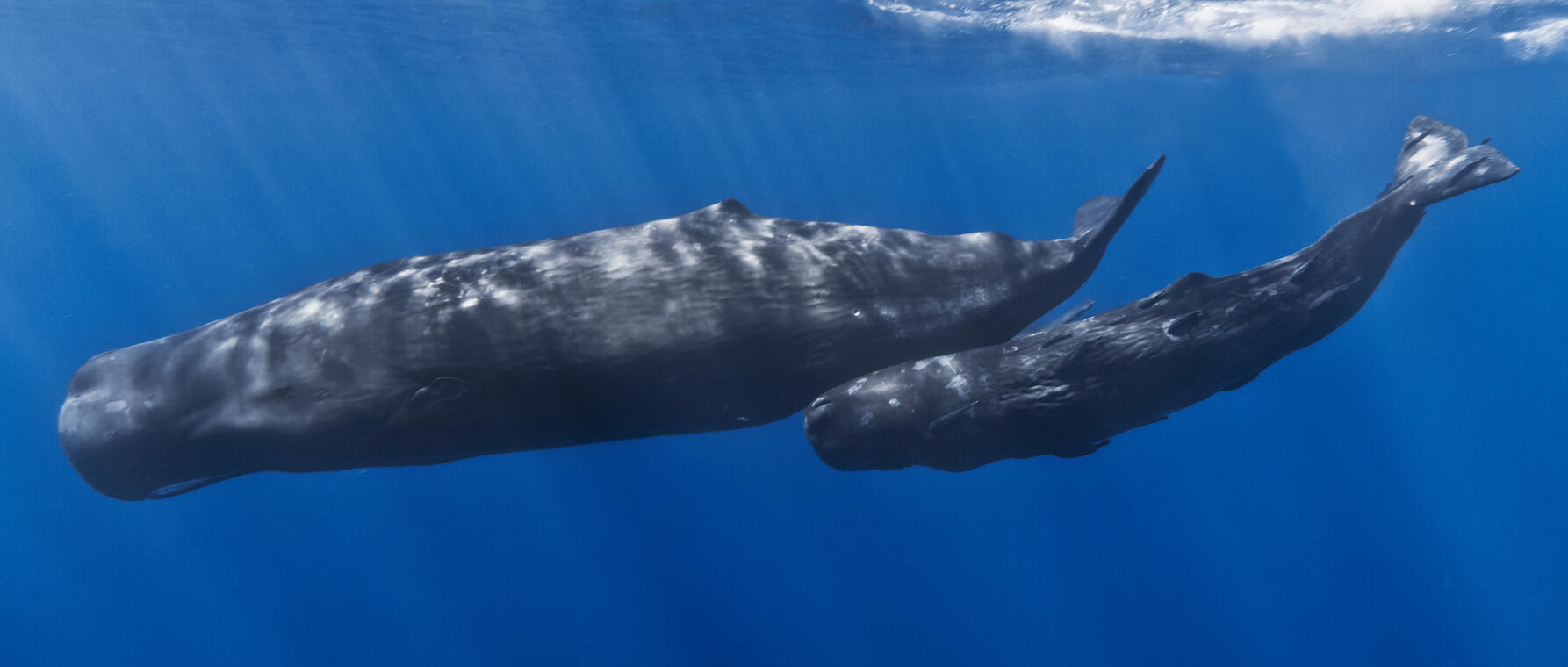
Gašper Beguš is an assistant professor in the Department of Linguistics at the University of California (UC), Berkeley, where he directs the Berkeley Speech and Computation Lab. He talks about how he and his colleagues gain insights into machine, human, and animal language learning; how his training as a historical linguist informs his current work on artificial intelligence (AI); and his time at Harvard as a Mather House residential tutor.
Building Baby Learners
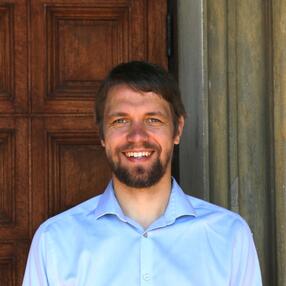
In our lab, we try to understand what is uniquely human about our language and how we can use that knowledge to better understand how artificial intelligence learns. Scientists have a relatively good understanding of what language is, how we learn it, and, to some degree, how our brain behaves when we communicate. We know much less about how AI learns.
We approach the task of understanding AI by building machines that learn more like humans do. Large language models are usually trained on massive amounts of text. Human babies, however, don’t learn from text; they learn from hearing spoken language and trying to imitate it. We created an AI baby language learner that learns by producing and listening to raw sounds of human speech. They never see text or labels. We even gave them representations of the human mouth and tongue so they need to learn to speak similarly to humans, limited by these representations of articulators. This might be the closest approximation of a human language learner using AI technology.
By using this artificial baby language learner, we can show that the stages and steps in language acquisition are very similar in humans and machines. We can also scan human brains and compare how, at the neural level, humans and artificial neural networks process language in similar and different ways. Our lab was the first to show that brain responses to speech are highly similar to machine responses in raw unchanged form. Understanding how machines are similar and where they differ from humans is crucial for effective regulation of AI.
We also developed techniques to understand how AI learns. This allows us to find patterns in data that we do not know a lot about. Human researchers are always biased by our biology. AI models, together with the interpretability techniques that we’re developing in the lab, can provide tools for finding patterns that we would have missed as biased humans.
One of the best testing grounds for this approach is animal communication systems, such as that of sperm whales. Sperm whales are an amazing species with many similarities to humans—they have complex social structures, the largest brains of all animals, and culturally learned vocalizations. On the other hand, their worlds are vastly different from ours and there is much we do not know about them. They communicate through a series of clicks called codas. We have no idea what the meaningful units are in their communication. We train our model on sperm whale communication and then we look inside the AI models to get clues about what the clicks are and other meaningful units.
It is exciting to think that linguists don’t study only human language anymore. Perhaps it’s the first time in history that machines can learn language so well that they become relevant to linguistics as well. And we’re discovering that there exist many analogs to human language in various animal communication systems. Studying language, we now have to take animal and artificial intelligence seriously as well. I strongly believe we can learn a lot from both animals and machines.
The Language of Community
I studied historical linguistics during my PhD work at Harvard Griffin GSAS. Historical Indo-European linguistics is the study of what the mother of all Indo-European languages—which include Latin, Greek, Sanskrit, and Hittite—sounded like thousands of years ago. To learn how to reverse engineer the mother language, I needed to spend several years carefully studying their philologies. My field was far removed from AI on the surface, but the things I learned turned out to be invaluable for the work I do now.
The highlight of my time at Harvard was my service as a resident tutor in Mather House. My wife and I were there for four years, and it was such an uplifting community. We met so many brilliant people and worked with so many awesome students. Some of them went on to successful careers in machine learning and are now my colleagues. A lot of Harvard alumni come to the Bay Area. Sometimes we get together with the former tutors and students who live and work around here.
My wife and I loved the residential college life at Harvard so much that we didn’t wait long to join another such community. I serve as a college principal (the equivalent of faculty dean) of Bowles Hall Residential College. A funny fact is that our college was built one year before the Harvard Houses. We can claim we’re the oldest in the nation!
Get the Latest Updates
Join Our Newsletter
Subscribe to Colloquy Podcast
Simplecast Stitcher