A Healthy Concern for AI
Zilberstein studies how cultures and organizations influence thinking about the benefits—and potential harms—of machine learning in healthcare
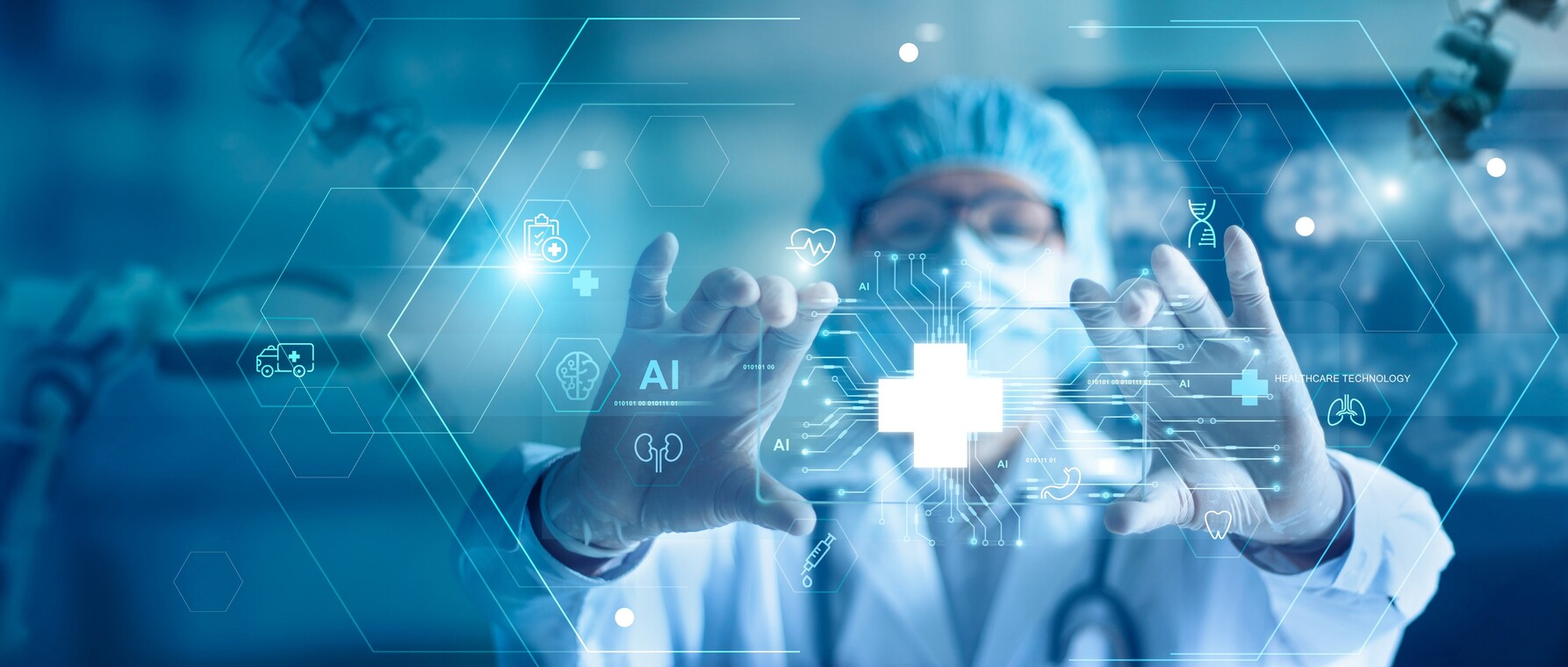
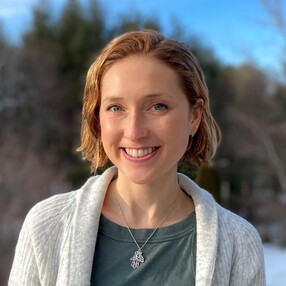
“Lucky” might not be the first word that comes to mind when you think of someone who lives with a debilitating autoimmune disease but that’s how Shira Zilberstein describes herself, at least for the decade she was on a treatment that kept her healthy. In 2020, though, her luck ran out, and her doctors struggled to find a therapy that could sustainably keep her condition under control. She spent much of the summer of 2022 in pain misdiagnosed with a stress fracture before she eventually found a physical therapist who successfully treated her for joint inflammation connected to her autoimmune condition. Today, she wonders if she might have recovered more quickly if her clinicians had leveraged the power of machine learning.
“Because we are sent to medical systems and doctors that see things in terms of their specialty, even with the best intentions and care, they cannot necessarily view everything about a patient’s health holistically,” she explains. “I think an algorithm may have been able to look at all my medical conditions together, associated different symptoms with different probabilities, and come up with a better and faster diagnosis.”
The healthcare sector increasingly uses machine learning or artificial intelligence (AI) for a wide range of tasks, from diagnosis and classification to genomics and drug discovery. But concerns about privacy, accountability, and, perhaps most of all, bias, present big challenges to implementing AI in a way that improves access to—and quality of—care. As a PhD student in sociology at the Harvard Kenneth C. Griffin Graduate School of Arts and Sciences, Zilberstein explores the way that cultures and organizations influence how research and development teams conceive of the benefits—and potential harms—of machine learning in healthcare. She finds that important stakeholders are often left out of the AI process, often by institutions with the resources to produce the most innovative technologies.
Minding the Gap
While much research focuses on machine learning products and their downstream consequences, Zilberstein’s work stands out for its focus on the process, according to Ya-Wen Lei, a professor of sociology at Harvard.
“The efficacy of AI projects often depends on effective collaboration between developers and experts with domain-specific knowledge, with each party contributing unique expertise and perspectives,” she says. “Shira’s work provides insights into the dynamics of these real-world collaborations, potentially pinpointing the conditions and mechanisms that foster better results.”
Using data from extensive interviews, ethnography, and textual analysis, Zilberstein studies three interdisciplinary labs that involve collaborators from computer science, engineering, statistics, medicine, and other fields. Her research reveals some of the tensions and trade-offs that arise in machine learning and healthcare projects. When negotiating ambiguous value decisions, for instance, research teams often default to the judgment of a senior clinical collaborator, giving them the responsibility of defining which technologies are worthwhile and which outcomes are acceptable.
“The clinician’s role is often to explain and recreate a narrative from abstracted bits of data,” Zilberstein says. “That’s important because a lot of the technology research and AI focuses on what these systems obscure—the black box—and less on the processes that make different aspects of algorithmic systems more concrete.”
Zilberstein tells the story of a bioengineering student in one of the labs she studies. Presenting his work to the wider team, the student explained that one of the AI models included the prescription of stool softeners as a variable for predicting treatment options in an intensive care unit. But a clinician attending the presentation explained that doctors often issue a standing order for stool softeners because a wide range of medications can cause constipation.
The fact that a small number of clinicians engage in the development of machine learning models builds bias into algorithms and also incorrectly represents the world that they’re trying to mimic.
—Shira Zilberstein
“His point was that, just because there’s an order for stool softeners in the system, that doesn’t mean that the patient is necessarily taking them or that it correlates with being on high painkillers, which would in turn correlate with a lot of serious medical conditions,” Zilberstein says. “So, using a prescription for stool softeners as a variable could bias the model. The engineering student had no idea about the relationship between all these variables. It was up to the doctor to explain in the moment their meaning by creating more of a narrative about how they come up in a clinical setting.”
Zilberstein’s story illustrates the way that AI developers can make inaccurate assumptions in the absence of a clinician’s perspective. But it also demonstrates the outsized influence of a select group of healthcare professionals who can understand and engage in machine learning projects.
"The fact that a small number of clinicians engage in the development of machine learning models builds bias into algorithms and also incorrectly represents the world that they’re trying to mimic,” she says. “It’s easy when you get a spreadsheet, to just think of it as numbers and labels, but there ought to be avenues—whether through something like a workshop, shadowing a clinician, or simply talking to a patient—to understand where those numbers and labels come from and what their implications are.”
Access to resources strongly influences the types of projects undertaken by different organizations—and who participates in them. Institutions need funding for salaries and tech-specific resources like computing power and software. They also need technical and clinical expertise. Finally, they need access to patient data or clinical data. The problem is that different types of organizations have different access to these resources.
Zilberstein tells of a lab based in a university computer science department. The machine learning team there has access to extensive computing resources and data through affiliation with a local medical center but lacks senior clinicians who can make judgments about usefulness and explain data elements when questions arise. Another lab based in a hospital works very closely with clinicians, but the group struggles to secure funding for expensive computing resources because the institution has a tight budget and its leaders aren’t convinced of the value of AI tools. Zilberstein says that these examples illustrate the gap between ideas, needs, and solutions.
“Organizations that have resources but a slim pathway for implementation often develop the most innovative tools,” she says. “On the other hand, an organization like a hospital or health center could implement new tools, but it typically has the resources to take on only modest and less impactful projects. These gaps affect the quality and diversity of the machine learning models created and put into practice by different institutions.”
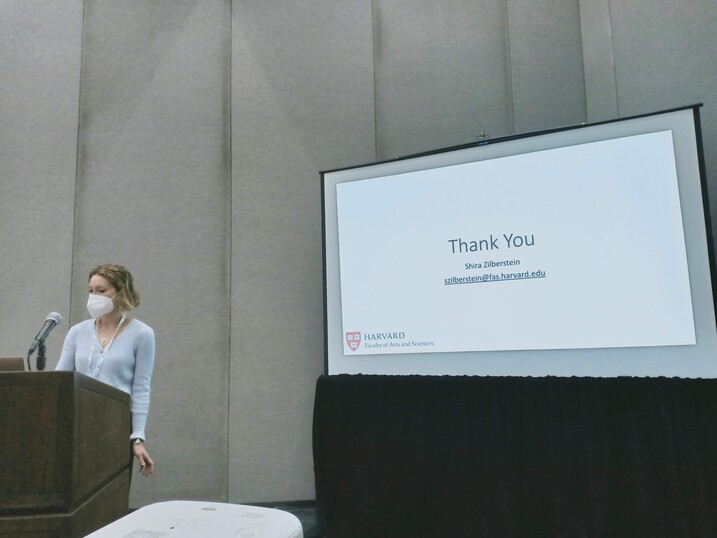
Broader Perspectives, Better Solutions
Still ongoing, Zilberstein’s research already offers some recommendations for improving the design and implementation of machine learning projects in healthcare. By integrating expertise in a way that is less hierarchical and allows direct access to clinical or user-centered implementations of AI, for instance, organizations can incorporate the needs and perspectives of diverse stakeholders and create better solutions. She acknowledges that discussions about how clinicians in less well-resourced areas, those with no background in technical fields, and patients in disadvantaged communities might play a role in design processes are “very preliminary.” Still, she says there are models to build on.
“The lab that had the bioengineering student and senior clinician in the same room is a good starting point,” she says. “That type of organization fosters collaboration, inclusion, and innovation in a way that produces more ethical and effective technology.”
I hope that Shira’s work will help governments and the various stakeholders think about evidence-based solutions to the regulation of AI and AI research.
—Professor Michèle Lamont
Part of a growing body of social scientists whose work recognizes the connection between upstream decision-making and downstream impact, Zilberstein says that she encourages AI researchers, developers, and policymakers to take a broad perspective. “Machine learning teams need to think through not only regulating tools after they’ve been created or trying to define narrow use cases where a type of tool would be acceptable, but also who gets to define social problems and solutions, and what types of artificial intelligence get made in the first place.”
Michèle Lamont, professor of sociology and of African and African American studies and, like Lei, one of Zilberstein’s dissertation advisors, says that her student’s work has implications for the regulation of ethical decisions around AI—an urgent topic that policymakers need to address.
“We have yet to institutionalize standardized mechanisms to govern what happens in machine learning—structures that could parallel the human subject institutional review boards that all universities have put in place,” she says. “I hope that Shira’s work will help governments and the various stakeholders think about evidence-based solutions to the regulation of AI and AI research.”
Zilberstein says her work is relevant for a wide range of healthcare AI users, patients, providers, policymakers, and advocates. But she also hopes that it can inform those in a wide range of fields who face the tensions that accompany competing priorities and conflicting incentives.
“The idea of neutral design and objectivity in theoretical science has been challenged over the past century,” she says. “It’s not just an issue for AI teams. It’s the broader story of science in our age.”
Get the Latest Updates
Join Our Newsletter
Subscribe to Colloquy Podcast
Simplecast Stitcher