Colloquy Podcast: How Reliable Are Election Forecasts?
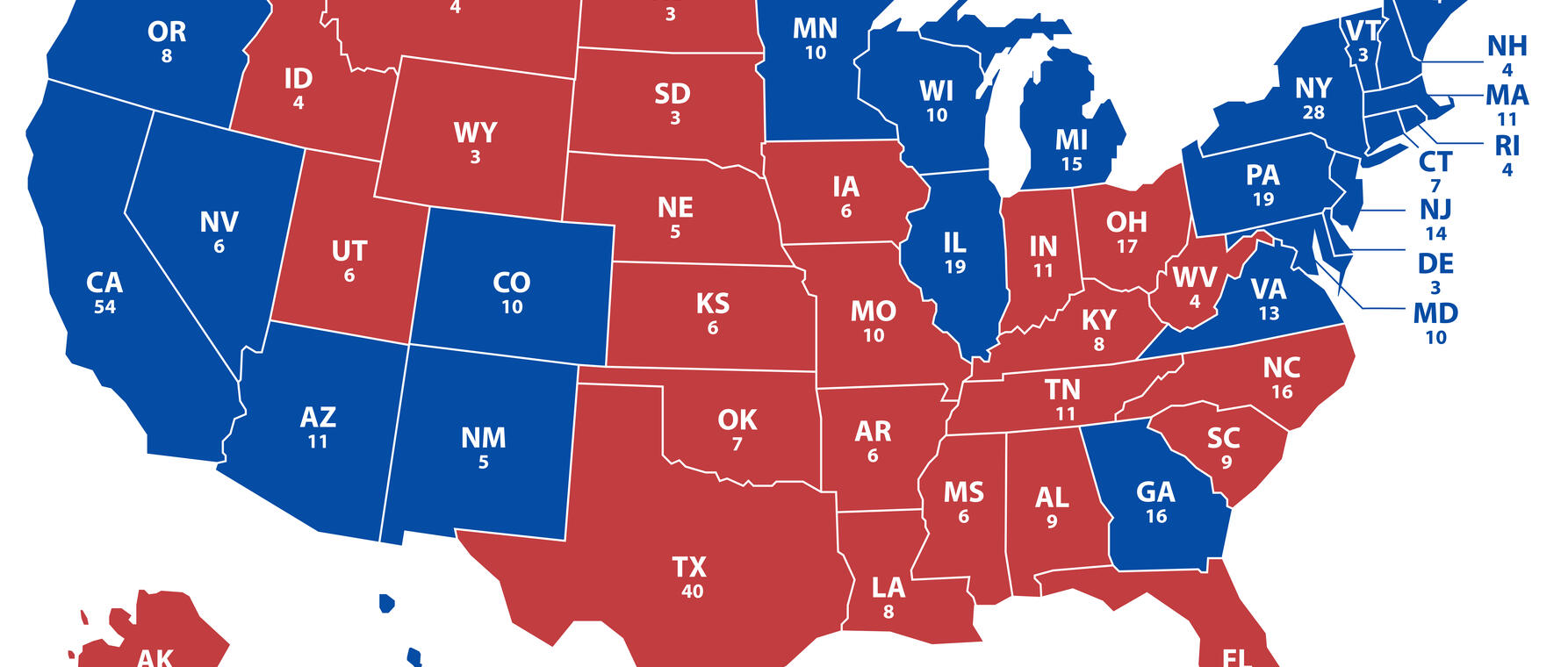
Just after Labor Day, American University Professor and Harvard Griffin GSAS alumnus, Allan Lichtman predicted a victory for Democratic candidate Kamala Harris in the 2024 presidential election. It was a source of some encouragement for Harris's supporters, given that Lichtman had correctly predicted the winner of 9 of the last 10 elections based on his historical analysis of campaign trends since 1860.
Despite his track record, Lichtman has been scorned by election forecasters like Nate Silver, who build probabilistic models based on weighted averages from scores of national and state-level polls. But are these quantitative models really any more reliable than ones that leverage historical fundamentals, like Lichtman's, or, for that matter, a random guess?
The Stanford University political scientist Justin Grimmer, PhD ’10, and his colleagues, Dean Knox of the University of Pennsylvania and Sean Westwood of Dartmouth, published research last August evaluating US presidential election forecasts like Silver's. Their verdict? Scientists and voters are decades to millennia away from assessing whether probabilistic forecasting provides reliable insights into election outcomes. In the meantime, they see growing evidence of harm in the centrality of these forecasts and the horse race campaign coverage they facilitate.
This month on Colloquy: Justin Grimmer on the reliability of probabilistic election forecasts.
This transcript has been edited for clarity.
Talk a little about probabilistic election forecasting. How does it work? How is it different from traditional polling? And how did it come to dominate public debate, drive obsessive media discussion, and influence campaign strategy as you write in the abstract of your paper?
So, let's just think about what a traditional poll is. So, a polling firm will go out and, one way or another, will try to get a sample of respondents. And they'll ask them, “How likely are you to participate in this election?” And then among the people who are likely to participate, they'll say, “Going to vote for Trump? Are you going to vote for Harris?” You run that poll and the result of that is going to be some estimate of what the vote share is going to be. Maybe it's for a state. Maybe it's for the whole country. That's going to be one component that's inputted into these probabilistic election models.
So, what's going on in these models is that they're going to aggregate together lots of different polling information, usually trying to construct some average of those polls. And it's also going to bring in other information, maybe about the approval rate of the president. Maybe it'll be about unemployment. Or perhaps it's about US involvement in foreign conflict. Combining that information together into a big statistical model, it's going to come up with essentially a probability of a particular candidate winning.
Usually what these models will do is they'll have some big model. And then they're going to do a lot of simulations based on these models and the statistical assumptions. And then after running those many simulations, they'll say that 600 out of 1,000 times Harris won in our simulation; 400 out of 1,000 times Trump won. And, therefore, we think there's a 60 percent chance that Harris is going to win this election. Along the way, they might also calculate things like the number of Electoral College votes they think a candidate is going to receive or the share of the popular vote. But the key thing is going to be that probability of a particular candidate winning.
Around 2008, [data journalist] Nate Silver really in earnest started putting these forecasts together, gathering polling data, and constructing these probabilities. And, notably, in 2008 and 2012, what Silver forecasted came to pass. He thought Barack Obama would win both of those elections. And he forecasted a large number of those states. Well, because of this, it became clear to, I think, a lot of news outlets that this was a way in which they could drive a lot of coverage about the election.
It turns out it's a nice summary of what might be happening. And it naturally creates a lot of stories. If the probability of a candidate winning an election moves from 55 to 50 [percent], well, maybe that could be a whole cycle of stories about what happened, why that probability changed, and what could be the origin. And so, I think the initial forecasts of Nate Silver and the attention that they seemed to garner really led to a lot of news outlets thinking this would be a good way to center their campaign coverage.
In your paper, you and your colleagues distinguish poll-based probabilistic forecasts from fundamentals-based forecasts, like Allan Lichtman's. What's the difference between the two? And how has each of them done in recent years?
So, poll-based is, as I've described, making an attempt to find all the polling that's been done and aggregate those polls together. Usually, they'll try to weight polls they think are more accurate so that you're not giving too much credibility or too much credence to a poll that hasn't done well in previous elections. And then based on that information, we'll try to project a potential winner.
Fundamental-based forecasts are forecasts that look at things like the unemployment rate or the growth in the economy in an election year. And they ask, historically, what's the relationship been between, say, first-quarter growth in an election year? And, ultimately, who's going to win the election or third-quarter growth? And who's going to win the election? And, historically, there is a nice relationship between things like growth and unemployment and who wins the election. It's not an iron law. There's lots of variability around that election.
So, in recent years, it's been hit and miss. So, obviously, Nate Silver seemingly is this soothsayer in 2008 and 2012. It looks like he's the best predictor around. 2016, it's a different story for these probabilistic forecasters. Some forecasters who started in 2016 only were on record as saying there's a 99 percent chance that Clinton is going to win. Nate Silver had a more modest prediction: 60/40 but still ultimately had made that forecast that Clinton would win. And I think many of us were surprised the day after the election to find that Trump was the winner.
In 2020, Nate Silver and some competing forecasts got it correct. They forecasted that Biden would win with some differences in the probability of victory. These forecasters also try to forecast things like midterms. In 2022, Nate Silver made a forecast that had a pro-Republican bias and thought there would be more Republican seats won than were. I think that's not surprising given where the polling data was. And I think, again, a lot of us were surprised at those election results.
We in our paper make a comparison across the two different kinds of models, not necessarily the Lichtman model but other political science style models. And what we find is that it's certainly true, based on things like vote share, that Nate Silver did better in 2008 and 2012. In the last two presidential elections, again, just based on those two elections, it looks like fundamentals might be doing better. But a core point of our paper is that if we really want to know who's better at picking the winner, it's going to take a long time to sort that out.
As you said, Nate Silver came to prominence in 2008 after his model predicted the outcomes of 49 of the 50 states in the presidential election that year. But you and your colleagues write of “predictive failures” and “growing evidence of harm” from forecasts. So, which failures? What harm?
I think, the clearest failure of these predictions is 2016, where the predictions that came out of these models were very confident to medium confident that Clinton was going to win. Certainly, the consensus view across the models was a Clinton victory. And that didn't come to pass. And so, they fundamentally got that election wrong. When we think about harm, it's interesting to think about how these models are being consumed by the public.
One of the things that seems to have emerged is that some people will confuse the probability of a candidate winning with that candidate's vote share. And so, the best evidence that we have from this comes from a paper by Sean Westwood, Solomon Messing, and Yphtach Lelkes, who examine in a set of lab experiments, where they can control a lot of the different pieces of information available—they show that participants in these lab experiments do regularly confuse the probability of a candidate winning with the share of votes that candidate is expected to win.
This is a problem they show in the context of these experiments because, as a result of this confusion, some people will disengage from these elections where they would have incentive to participate to help their side win. Translated to the real world, that means that there's a risk that people consuming these probabilistic election forecasts may conclude the election's already decided. It's a foregone conclusion. I don't need to participate. I can just go about my day. I don't need to take the time out to vote. And I think that's a real mistake. I think that would be a real harm if these forecasts were causing people to disengage from the political process.
I'll add just one other note about a different version of harm. Another colleague of mine, Eitan Hersh, has a book called Politics Is for Power. One of the points he makes in that book is that people should participate in politics and actively pursue what they want to see by active engagement. The risk with these probabilistic forecasts is it continues this horse race coverage of politics so that people are relieving their anxiety by consulting these probabilities, rather than relieving their anxiety by knocking on doors or calling friends or advocating for the candidate that they really want to see win. And insomuch as it's channeling effort away from the actual practice of politics, I think that's a concern.
The concerns you've mentioned about the impact of probabilistic forecasts on political participation—it seems to me that you could say them about polling in general. And if that's the case, should there just not be any polls?
I very much like free speech. So, I would not advocate for any sort of ban on polling. I think a lot of this comes down to responsibility at media outlets, journalists, and other individuals who are producing these assessments. I think polls can be very valuable. In fact, there's an argument to be made. They're a core component of what helps us be a responsive representative of democracy is that we have polls. Politicians can know what people want. And they can be responsive to that. So, I certainly wouldn't want to advocate for getting rid of polls.
What I do think journalists could do a much better job of and what forecasters could do a better job of is appropriately framing their analysis. They can offer a characteristic of what the current state of the race is. They could say, “This is where the current share of votes are. This is who's currently supporting the candidate or not.” I think it's another thing entirely to say, “This is the probability of this candidate winning.” That's a big difference from what's going on in previous polls.
And those probabilities resonate with people in a different way. If you're telling me it's a 95 percent chance my candidate's going to win, that's different than you telling me that my candidate is up by a lot in a particular state. So, I think that probability is a real point of distinction. And I think as with many things in life, it's a question about responsibly using them rather than the wholesale elimination or regulation of them.
The core of the argument in your paper seems to be that we simply don't have enough data points to determine whether these forecasts of presidential elections are accurate or at least more accurate than random guesses. Some of that has to do with the infrequency of elections. Some of that has to do with the nature and the quantity of information from the state results. Can you unpack each of these criticisms?
As you mentioned, at the very heart of our argument is that there are simply not enough presidential elections to evaluate these forecasts.
So, here's the thought experiment that our paper is really based on. Let's suppose we have two rival forecasters. One forecaster is fitting a very sophisticated model. Another forecaster, unbeknownst to everyone, is really just flipping a coin. Now, they may put a lot of window dressing on what that forecast looks like. They may write some elaborate scheme that tells them how they're making their choice. But really, at the end of the day, they're just secretly flipping the coin. The question we ask in our paper is, how long would we need to observe these election forecasts in order to differentiate these two forecasters to reveal that one forecaster is the sophisticated forecaster with lots of information like we see from Silver? Or is it someone who's just flipping the coin?
And so, our calculations are fundamentally focusing, at least at first, on the national winner, which is the big thing that forecasters want to pick. They want to be able to tell you who is going to win the presidential election. And when we focus on those, it turns out, depending on how much more skilled the real forecaster is, it can take anywhere between decades to centuries because we just need to observe enough election results in order to be able to differentiate the two.
Every once in a while, the coin-flipping forecaster—or half the time probably—the coin-flipping forecaster is going to agree with the real forecaster. And we can't tell them apart in those years. It's only in the years where there's some differentiation and the skilled forecaster comes out on top that we'd be able to tell the two apart.
Perhaps people can use information from the states in order to do a better job of evaluating these forecasts. I think there are two important facts there. So, first, it is the case that we could require forecasters to make state-level results. And we could say we only want to consider forecasts that are picking an overall winner and then give us something like state-level vote share. But performing well at state-level vote share is different and does not imply that you're doing a good job of picking the winner.
Suppose I have a forecasting model and it is superb at nailing the vote share in California and Wyoming and in North Dakota, these sorts of states, very lopsided states, Alabama. It is going to get this down. We know DC very precisely. And then we would say, wow, this is an excellent model. It's done very good at vote share. But, fundamentally, to pick the winner, we need a very precise estimate of vote share in the small set of swing states that are going to determine the winner.
So, even if you had done well at vote share in 2016 or 2020, at the end of the day, the question is, do you get Wisconsin, Michigan, Pennsylvania, Georgia, Nevada, Arizona, correct? And if you don't get those correct, it's not going to matter that you nail to the wall the California vote share or the Alabama vote share. You really have to get those states right.
And so, that's how you can have this breakdown between the state-level results and picking the overall winner. And so, it would be, I think, a mistake to rely too much on those state-level results. Really focusing on this national level is how we could differentiate someone like Nate Silver from someone like Allan Lichtman to someone who's just by happenstance making these sorts of forecasts.
You've gotten some pushback from Silver on social media. He calls academics slow compared to the efficient market and says they can't model. So, what is he missing?
I think the big point that we're making is that you evaluate a forecast, at least its historical track record, on the events that you are forecasting. If I say that there's going to be rain this evening, I think any reasonable person would evaluate that forecast based on whether there was, in fact, rain this evening. If I'm going to make a claim about someone who's going to win the presidential election, I will evaluate that based on who wins the US presidency. And academics and private sector and everyone else are getting that information at the exact same rate. It's going to be every four years on the first Tuesday after the first Monday in November. Well, we'll get the votes in and then, hopefully, shortly thereafter know who's going to be the winner.
So, everyone's subject to that same rate of learning. We could add in different assumptions about how these other forecasts feed into our evaluation. But, fundamentally, if we're going to make the claim, “I'm good at picking the US presidency,” you would want to see results from picking the US presidency. So, that's what he seems to be missing.
The data journalist G. Elliott Morris, the head of ABC's 538 election forecasting team, had a thoughtful critique of your paper. He wrote, "I think the problem is that they're evaluating each election outcome as binary. But some elections have more information than others. Predicting 1984 or 1996," which were very lopsided elections, obviously, "as a coin flip would be ridiculous. The key here is that forecasters predict the popular and electoral vote margins, not just the winner." So, how do you and your colleagues respond to Morris's points?
There are a few points to be made here. We show in the paper that even if you want to distinguish between rival forecasters—things like 538 or Morris's old model, which was at The Economist or other prediction models—in that realm, even if we're looking at something like Electoral College vote, that's going to take a really long time because these models are all so similar, you would have to see some differences across these models. That's going to take many, many elections. But I think there is this same basic idea.
Even if we wanted to use something like margin—and we think if we get the margin correct, we're going to be better at picking the winner, or we think it's about vote share and if we get we do a better job at vote share, we'd be better at picking the winner—the way we would evaluate that claim, at least historically, is we'd say, “Well, is it the case that our models that are better at predicting vote share, better at predicting the electoral college, are also more accurate in selecting the winner of the presidential election?”
And what you confront immediately when you realize that is that you will learn that relationship at the same rate that we're all learning this relationship, which is once every four years, we'll get the winner of that election. And so, we might say, data free—before I look at data, I just prefer models that have been more accurate historically on the electoral college or more accurate on vote share. And I think that's fine. I think we'd also want to be very clear that we don't know that those models that have been more accurate on electoral college or vote share historically are any better at picking the winner than a model that has more inaccuracy in those other targets.
Morris also writes that political professionals, journalists, and gamblers are going to make probabilistic forecasts one way or another. Fundamentals-based models exist. Polls exist. Given that this information is going to be combined in some way, he says, “I don’t think there’s any shame in trying to do it well.” So, does he have a point? Are we stuck with probabilistic forecasts? And if we are, should we at least try and go about them as best we can?
Just as a matter of logic and historical record, there's absolutely no reason forecasts, as they're currently instantiated, need to exist. They didn't exist for a long time in US political history. They could go away and my guess is we'd probably be OK. In fact, I think some of the evidence I've already discussed would suggest that we might be better off if they did go away. And so, their usefulness is a question that I think could still be debated, hardly settled science but certainly a debate worth having.
I do agree with Morris that, given that it would happen, there's a way in which we could convey the information out of these models better. I think better transparency about what's going in, better transparency about what conclusions are based on the data that's being inputted, and what conclusions are based on assumptions would be really helpful for the public in informing how much they should trust these forecasts. And I think, certainly, it would be helpful for knowing how journalists should approach them.
And then the last thing Morris said is, “Well, we should do it well.” And that's an appeal that resonates with me. It's like, “Well, we're going to do something. Let's work hard at it. Let's try to do it as well as we can.” But I think it's useful for us to think about what doing well means.
And so, certainly, we can think about applying reasonable statistical standards when crafting our models and being sufficiently transparent about the assumptions that we give and, even as some forecasters will suggest, looking at the results and making sure that they pass some face validity check. Those things are all reasonable. And I agree with Morris. You want to engage in that effort.
But there's a different way to think about what it means to do well. And that would be, “Are these models with all these reasonable assumptions and best statistical practices in face validity—are they any good at picking the winner?”
And I don't mean to sound repetitive here. But to do that, that's going to take a long time to assess. And so, whether or not the best practice statistical procedures manifest in being a very accurate election forecaster picking the winner will take a long time to figure out. And maybe we'll never know.
All right, so, finally, come on now, who do you think is going to win in November?
That's a hilarious question. In March, if you would have asked me, I would have said, this is going to be a very close contest that will come down to who will win a collection of swing states. We've had one candidate survive a near-assassination attempt. We have another candidate totally drop out of the race. We had two historic debates really unlike any other we've seen in American political history, with wild accusations from both candidates. And as we sit here today, I think the best summary of what's going to happen is this is going to be a very closely contested election. And the winner is going to be whoever is going to be able to secure this small collection of swing states.
Get the Latest Updates
Join Our Newsletter
Subscribe to Colloquy Podcast
Simplecast