Colloquy Podcast: A Glide Path to Getting the Most from ChatGPT

Tufts University Professor James Intriligator, PhD ’97, a human factors engineer, says that GPT is not a search engine, although many of us use it that way. It's more like a glider. It can take us to great knowledge and help us explore new territory. But we need to steer it smartly to get where we want to go. In these journeys, our own curiosity is the wind beneath ChatGPT's wings, the force that unlocks AI's almost limitless potential. In this episode of Colloquy, James Intriligator maps out a flight plan for GPT glider pilots. He says the questions we ask the large language model can take us through transversal spaces that cross many different areas of knowledge. And he's got some important advice for steering it through these domains to get better answers.
This transcript has been edited for clarity and correctness.
First of all, just as a background, what is a human factors engineer?
It's basically the intersection of engineering, psychology, and design. So if you're trying to make a new app for your phone, you might want to talk to a human factors engineer to think about things like human memory and attention and all the psychological aspects of design. It's the intersection of physical, cognitive, and emotional design for everything.
Before we get to the right way to interact with ChatGPT, let's talk a little bit about the wrong way. We're so used to googling things. We've been doing it for a couple of decades now. But you say that's not the way to understand large language models. Why should we not think of ChatGPT as a kind of souped-up search engine? And why should we not use it that way?
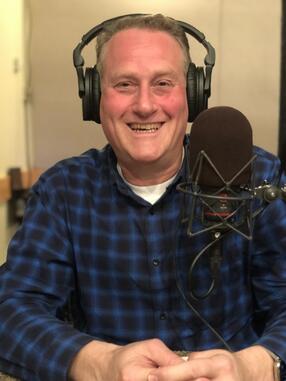
I think part of the issue here is that OpenAI when they designed the system, made it have an interface that looks like a search engine. There's a nice box there that looks like a search box. And in the world of human factors engineering, we talk about the mental models that people have. So everyone has an internal mental model of how a system works, whether it's part of the human body or part of a computer program or an app you're using. And the issue is often the mental model that's evoked is a function of what the interface looks like.
So if it looks like a duck and quacks like a duck, it's almost that kind of thing. But in this case, it looks very much like a search engine. And so people are tempted to search.
But it's quite different. On the back end, it's not that ChatGPT is ever going and finding information and having a static database of answers that it gives you. It's generating everything it ever gives you afresh. So it's more like a creative synthesis machine.
If you're looking for facts, for instance, probably you wouldn't want to go to ChatGPT. It wouldn't be the first place you'd go. It's not good at facts.
A lot of my colleagues say don't ever use it to write papers because it'll just make up references. And that is true. There are other systems out there that do much better at that.
But, in general, it will make things up. It's a brilliant creative synthesizer. I mean, I think of it almost like an oracle of ancient days or a god in some ways. I mean, it has seen everything humans have ever written. It's been trained in everything humans have ever written. Every book, article, blog post, anything you can find on the web, basically, it's read.
And, of course, even if you are a very powerful godlike being, you can't remember all of that. And so ChatGPT has been forced to compress a lot of that information and get the gist of some things here and there. And that's both a blessing and a curse. I mean, it's been able to abstract away and understand some of the deeper issues and deeper themes and messages and meanings embedded in the texts. But it's also then lost a lot of the specifics.
So if you ask it about a current theory in medicine or some area of science or culture, it'll have the sense of what that's about. But it won't have the right details. If you start asking it for details, it'll just start making things up because it thinks it knows. And that's where you have to be a little bit careful.
In an article you wrote for The Conversation last year, which actually inspired me to have you on the show, you equate interacting with ChatGPT to gliding. So what do you mean by that? And how does that metaphor help users to get a better understanding of the way ChatGPT works?
There are a lot of different ways you could come at this concept of gliding. But I guess the easiest, perhaps, is through an example. I was talking about ChatGPT with a friend of mine and, actually, another graduate from Harvard, Bryan Reynolds. He's now a professor at UC Irvine. And we've done a lot of work on transversal analysis, which is another area of interest of mine.
Anyway, recently, we were together. And he was interested in how ChatGPT might work. And so he decided to give it a test and give it a question. And his question was, OK, ask GPT who's the greatest motocross racer of all time.
And I said to him, that's a very specific, factual question. And it's a bit ambiguous what you mean by greatest. And he said, I know, I know. That's why I want you to ask ChatGPT because I'm going to show you it's an idiot. It'll just say something that's absolutely wrong.
And I said, well, you're coming at this the wrong way. We don't want to just ask it for an answer. We want to use it as a mechanism for exploration, let's say.
So we took a step back and decided to start by asking ChatGPT, there's a sport out there called motocross. I'd like you to think about every aspect of motocross and come up with eight clusters of skills, expertise, knowledge, and wisdom required to be a great motocross racer. And it did.
One of the little tricks that I discovered on my own is that if you ask it to cluster a space, it really forces it to do a deep dive and analysis of the space. I like to think of it as forcing it to make its knowledge that's implicit, explicit. So it knows everything but it knows it all at a very coarse level. If you ask it to start clustering a space, it forces it to make it much more high-res and activate that part of its knowledge base, if you will.
So we went from there. That was the first step on our glide—to start exploring issues around what it takes to be a motocross racer. And then we dove down into each of those things.
So we talked about having to be part of the—well, let's say, the technical advances in the field. And I asked it to tell me more about that, and explored technical advances, and explored a few of the other clusters just to make sure it really started to understand what it means to be embedded in and excellent at motocross. And then we navigated through this space until we finally got to the question of, OK, considering everything we've been discussing around the cultural phenomenon, the technical, the performance, the mindfulness—it went through some fascinating clusters.
Then I asked it, OK, so considering all those things, can you put together a list of some of the greatest motocross racers? And my friend Brian was blown away. It did a fabulous job. It has very nuanced understandings as long as you come at it the right way. Each question you ask ChatGPT should be priming it and preparing it to get to a deeper, richer, better answer to your ultimate question.
You've done a great job explaining this idea of having a more in-depth conversation with GPT and the notion of asking it to group things into clusters and to get it to think more deeply. So how is that like gliding? Why did you pick that metaphor?
I guess, I mean, the way I think of it is, if you imagine concepts in space or all of knowledge laid out in this billion-dimensional space, what you're really doing with ChatGPT is you're exploring that space. If you want to come up with a new idea for how to engineer a bridge to be more resilient to winds, it means you have to think about what happens when winds hit bridges. Think about new materials for bridges. It’s like
any time humans are thinking about stuff, we're actually moving through these conceptual spaces ourselves. And it's very much the way ChatGPT has been built, in a sense. It's learned everything about the world. All of its knowledge has come from our artifacts.
Us, as humans, we've written stuff. And that's how it's been trained. And so it has, in a strange way, an encoding of knowledge and reality very much like ours because it's been trained by us. And so we tend to have linkages between ideas.
From a psychological perspective, we have lots of different realms of knowledge. And each of them can be deeper, richer, and interconnected. And the idea of exploring those spaces to me somehow—maybe it's just me, but it feels very much like a spatial metaphor works pretty well, that it's exploring different parts of space.
You used the word transversal, which is something that you talked about in your article, as well, these transversal spaces where you're gliding through these different fields of knowledge, which was another way in which I thought the gliding metaphor was really appropriate. Up top in the intro, I was talking about how our curiosity, in some way, is the wind beneath the wings of the glider. But it's not all us. We're in this interaction with GPT. And it throws this different information or these different ideas at us, which hopefully sparks our curiosity, almost like the wind moving a glider in one way or another and taking us in different and hopefully interesting new directions. So I'd love you to talk about this idea of transversal space as a place where design and innovation thrive.
Over the last, I guess, 5 or 10 years, I've been thinking about transversality in design. And that, to me, is now the clearest case. If you're trying to design something new, let's say a new cup or mug, or coffee cup, you might want to start to approach it from lots of different perspectives.
So, if I'm trying to make a perfect cup for you, I'd like to understand what your favorite color is. The color of the cup will be a function of what matters to you.
In terms of the size of the cup, if I'm designing it for you, I'd need to know the size of your hand. If I'm designing it to be a product sold in stores, I need to know the size of the average human hand. This is where human factors come in. That's the kind of stuff human factors think about.
But there are a lot of other dimensions you could think about if you're trying to design a cup. So we talked about the color, the size. We could think about the aspect ratio. We could think about the texture and the material. If you're making a cup for people who are going to climb mountains, you might want it to be ultra-light.
You might care about the cost. You might care about the brand or the logo. If you're interested in social justice issues, maybe you should have a logo on it that conveys some kind of cause, for instance.
And the specifics of the design really depend on which dimensions matter to you. If you're really focused on making it cheap as chips, as they say, a super cheap, generic mug, then that's fine. You won't care about sustainability issues, for instance. But if you're trying to make a cup for Patagonia, they really care about sustainability. So that dimension will be really important.
So the idea here is that any object sits at the intersection of all these different dimensional constraints and opportunities. And so along comes ChatGPT. And it turns out to be a perfect little partner for doing this kind of transversal design. In fact, in my classes on product design, I'm now teaching it as transversal design. And the idea is that it's up to the designer to take control over the flight path.
So let me put you in the pilot's seat for a moment. And maybe you can take us through a glide. What if I wanted to use the gliding method to work with ChatGPT to come up with an audience growth strategy for a podcast like [Colloquy]?
I mean, I guess what I often recommend to my students, for instance, when they're starting to explore these kinds of spaces—and not just students, but everyone—is to understand who your customers are. So in this case, I'd maybe begin by asking about the kinds of people who listen to podcasts. So, hey, ChatGPT, currently there are millions of podcasters out there and millions of people listening to podcasts out there. I'd like you to think about all the different kinds of podcast consumers out there and cluster them into eight clusters of types of podcast consumers.
Describe each one in detail. Give it a name. Give it a strapline. And offer some thoughts on sub-clusters as well.
If I had it up and running, I could just do that. And it's amazing because within two seconds, it'll start just giving you answers. There are eight clusters of podcast consumers. They are these types, these types, these types, these types.
Now, again, when I do these kinds of glides, I actually don't even really read what it says because I don't really care that much about the specific answer it's going to give. I just mostly want it to remind itself about the nuances and depth and intricacies of the target customer, in this case. I usually skim it and look at the clusters.
It makes sense. You have people who are commuting, that makes sense. People are trying to learn new skills, and that makes sense. People who are passionate about a cause—I'm making these up. I don't know what they would be. But my guess is it'll be something like that.
And if you see it's got something wrong, then you know you've probably asked the question wrong. Sorry, I meant to say people who regularly consume podcasts not just—or whatever. And so usually I skim it. And I know now it has a deep understanding of podcast consumers.
And now for this specific case, I would say, OK, now I'd like you to think about all the research, professors, skills, resources, and expertise available in the Harvard community, both literally within Harvard and also more broadly within the alumni network, et cetera, et cetera, and identify 10 clusters of expertise, interest, or excellence that might be of interest to the general public. And it'll go ahead and give you some examples or some clusters for those kinds of things.
And then you could start looking at them and even start to ask whether there are particular clusters within the Harvard areas of expertise that might be interesting to specific podcast consumers. You could even ask it to examine all combinations of podcast consumers and areas of expertise and come up with eight ideas for novel, innovative, and likely-to-succeed new podcast formats, episodes, et cetera. And it'll do it.
And it might not be good. But, often, you'll find that it'll have—maybe it'll have four or five things that you've already thought of or tried. And often, it'll have a couple that are like, oh, that's an interesting idea. I hadn't thought about that. Let's dig into that.
And then you just glide deeper. You say, OK, cluster four above talked about medical advances that might be of interest to—I don't know. I'm making this up, so it's a little hard on the fly. It'll come up with a cluster there.
And you'll say, OK, that cluster is quite interesting. I'd like you to think about that cluster some more. I'd like you to analyze it from many different perspectives. And I'd like you to come up with six specific sub-clusters within that type of podcast that might be successful, interesting, et cetera, et cetera. And it'll do that again.
And you might then find one of those sub-clusters that looks juicy. And you can say, OK, that's a fascinating area. Can you lay out the specific format for that kind of podcast?
What would it be like? What kinds of guests would we have? What kind of questions would happen, et cetera, et cetera? And you can keep going deeper and deeper.
When it's building you these clusters, how do you know that it's not just making it up, that it's inaccurate?
It probably will be making up—I mean, I like to say, it's always making up everything. That's all it ever does. It is just a text generator. So anything you see is just being generated on the fly.
I have an image still in my talks where it's a little wind-up robot. And I have an image where it's a wind-up robot going along just leaving footprints that are text. And I like to think of it more as a kind of a hypothesis generator. You shouldn't ever take anything it says as fact.
Right now, most of the concepts I'm playing with are more at the conceptual level. When it comes time to reduce it down to practice, so to speak, then I'd actually have to start looking. If it's vital that I’m actually using clusters that are meaningful, or if I want to give a report back to someone who's hired me and say, here are the six clusters, I wouldn’t ever do that just based on ChatGPT saying it. It’s funny, I mean, it seems like the best skill that you can have is to be a really good interviewer.
This brings up another question that I have for you, which is, do you have concerns about how this will change our relationship to knowledge and the way that we learn? I mean, obviously, there have been concerns ever since—I don't know whether it was—I think it was Socrates who thought you should never write anything down because that'll be the end of real learning and knowledge and mastery. So we've always been freaked out by new knowledge technologies. So do you have a concern, though, that we won't ever really know anything or learn anything anymore? Or are you feeling like, no, actually, to use this well, you're really going to have to know a lot?
Oh, there's a bunch of really interesting, fabulous observations and questions in there. Let's see, I just wanted to—I didn't want to lose one thing that you had mentioned, which was to be good at using ChatGPT, you probably have to be a good interviewer. And I think that's definitely true.
I'm actually working with Tufts to develop a new course on Prompt Engineering for English Majors, is what our working title is. And I like to imagine this strange future where 10 years from now, it may be that someone with an English degree is actually getting offers of $300,000 a year. And computer scientists, they're a dime a dozen. Machines can program as well as a human can. But you really need the English philosophy.
I mean, it will be the day when the humanities are actually going to finally make the comeback and get the pay they deserve and the recognition they deserve because it really is amazing. I think it will be people who have a background in English, anthropology, sociology, and things like that. People who understand how to work with words and how conceptually to play in spaces—those are the people who are going to really excel at using LLMs, ChatGPT, and generative AI, in general. So I think that that's an interesting direction for future thought.
In terms of the skills, knowledge, expertise, let's say, required to be a great scientist, engineer, author, construction worker, anything, I think you're right that there will be this need for a totally different kind of knowledge on top of the basic knowledge. So if you're going to be a great biologist, you definitely need to understand genetics, and you need to know how biology works and all kinds of stuff. But you also need to have this whole other skill set to be able to use generative AI to help you find new avenues for inquiry.
Consider unexpected or novel ways of combining interdisciplinary insights for instance. I think that starting to train people in terms of how to use ChatGPT and how to use it productively to magnify their intelligence, creativity, and innovation, I think that's an urgent need. And how it's going to happen is unclear to me. I'm a bit concerned about that myself.
Well, that's a great jumping-off point because I want to ask you to step back and think about larger ethical issues. And so when I hear you talking about the glider approach to ChatGPT, what I hear is that this is really going to be most fruitful to imaginative, creative, thoughtful people who are going to have a conversation with this technology. My concern is that that's not the way markets work. That's not the way our society works. And so I'm wondering if you worry, or maybe you already see companies, people, and organizations using this technology as a shortcut rather than maybe a longer but more fruitful pathway to better ideas, better knowledge, and better tech.
I mean, I guess the way I think about ChatGPT is that it can help anyone do anything they want to do better, that’s how I often put it. And there are pros and cons to that. The use case that we've mostly been discussing today, those use cases have tended to be around new ideas, new directions, new products, new inventions, all that kind of stuff.
But it also is fabulous at helping you do just mundane tasks like if I have to—what's a good example? Well, I've recently had it start writing some first drafts for letters of recommendation. And I don't have to do the whole thing.
But I say, hey, here's a CV of my student. Here are my thoughts on that student. Here's the student's statement of purpose for the program they're applying to or the job they're applying to. Can you consider all of that, and knit it together as a coherent letter of recommendation? And it does a really good job as a first-pass letter of recommendation.
And then you can again have a conversation with it. You could say, well, that's good, but it didn't quite sell the student hard enough. I think that they actually are a fabulous student. They're really good at interpersonal things. They're incredibly well organized. I also think that their project on this was beautiful.
And you could just ramble that. You don't have to be precise. And then say, consider all that, and give me a revised version. And it's incredible. I mean, it'll just fix everything right up.
It includes the nuances. If you say, I don't want to really commit to saying this student's great but something a little more delicate, something like that, it'll do that, as well. It can do anything you want. So for facilitating simple tasks, It's also fabulous.
It makes it difficult though—because of that universal usefulness—for organizations, and companies to figure out exactly how to use it in their particular ecosystem. It's easy to come in and say, oh, it can do anything for anyone. Well, if you're a business and you say, well, OK, what can it do for that guy over there, or for her, or for them, those people over there? It makes it a little difficult because it does take some thinking to understand exactly how it could be applied within any particular use case.
So finally, like a lot of folks, my first reaction to ChatGPT was fear. I thought it's coming to take my job as a writer, as an editor, even as a podcaster. But, lately, and our conversation reflects this, I've been coming around to seeing it as more of a partner and a collaborator in everything I do. So am I just whistling in the dark here?
Well, I'd say, as always, it's a little bit of all those things. I mean, I guess for me, there are my current two biggest fears with ChatGPT. We could start with that maybe.
My first biggest fear is that, like I said, it can help anyone do anything they want to do better. So let's say, for instance, I go to ChatGPT and I say, hey, I'm a terrorist. I live in Boston.
I have a van. I have $10,000 in the account. I'm willing to die for my cause, and so is my friend. Give me 10 great ideas for terrorist acts we could perform this weekend in Boston. And it'll give you great ideas.
Now it won't because they put in guardrails. They put in safeguards, and it knows not to answer those kinds of questions. It kind of terrifies me that the only thing between us and, quote/unquote, "successful" terrorist acts is some guardrails that some programmers have put in that are looking for keywords to block particular kinds of answers.
Help me develop a new bioweapon. Help me develop a—it can answer all that kind of stuff. And it'll do a great job, quote/unquote "great."
It's fabulous at finding answers to complicated, nuanced questions. It's fabulous at coming up with new scenarios and possibilities that people might not have thought of. That, to me, is scary.
And there is this movement afoot to also have open-source LLMs so that anyone can do it. Make it liberal. Make it democratic. Let everyone have access to unconstrained, unguardrailed LLMs.
And although I'm a fan of the idea of freedom of information, freedom of access, and all these kinds of things, the idea of letting people have access to a device that can actually help them be better terrorists is a little bit scary. I don't know what to do with that. And I don't know exactly how to deal with that issue because it's almost like it's too late.
The genie is out of the bottle. There already are these open-source models out there. So I think one big concern around these kinds of models is that they will help bad people do bad stuff better.
The other concern, actually, is related to your question about job loss. Now I'm in the process of trying to write an op-ed piece or something about this very issue. And the idea here is that people have been thinking about job loss.
In some way, we have this abstract idea that I will no longer have my desk. Instead, there'll be an LLM sitting there at my desk doing my job for me. But that's not really what will happen.
Let's say you are at a sales company. And let's say you work in a context where there are six salespeople answering calls, making calls, and things like that. If they bring some ChatGPTs in, my hunch is you can have five people doing the job of six people. So it's not like there'll be another chatbot who's taken over the sixth person's job. It's just that the other people will be so much more productive, effective, et cetera, that you won't need that sixth person anymore.
And the interesting and scary thing to me is that that's going to be true from a systems perspective. I was saying how human factors, we like to look at systems. That'll be true at every level of an organization.
So you don't need quite as many salespeople at the bottom, which means the next-level-up managers are happy. They've saved some money. Ta-da, that's great. They have a little bit more money. Maybe they could pay the other people more, et cetera.
But at their level, they'll be maybe five or six mid-level managers. And now the person above them will say, oh, we actually don't need five or six. We only need four of those people.
And when you think about it as a systems effect, it's going to basically lift all the profits and power to the top of the pyramid. And as I'm sure many are aware, income inequality, wealth inequality, and power inequality are some of the biggest problems in our society. And my fear is that these technologies will become real magnifiers for that phenomenon, that the rich will get richer at an unprecedented rate. They won't need to hire as many people. People will have trouble finding jobs.
I think this, again, is another factor that, to me it seems inescapable that given this technology, we're going to need some kind of universal basic income because there won't be as many jobs for them to do. There won't be as much need for humans.
The Colloquy podcast is a conversation with scholars and thinkers from Harvard's PhD community on some of the most pressing challenges of our time—from global health to climate change, growth and development, the future of AI, and many others.
About the Show
Produced by GSAS Communications in collaboration with Harvard's Media Production Center, the Colloquy podcast continues and adds to the conversations found in Colloquy magazine. New episodes drop each month during the fall and spring terms.
Talk to Us
Have a comment or suggestion for a future episode of Colloquy? Drop us a line at gsaspod@fas.harvard.edu. And if you enjoy the program, please be sure to rate it on your preferred podcast platform so that others may find it as well.
Get the Latest Updates
Join Our Newsletter
Subscribe to Colloquy Podcast
Simplecast Stitcher