Colloquy Podcast: Is AI Coming for Your Job?
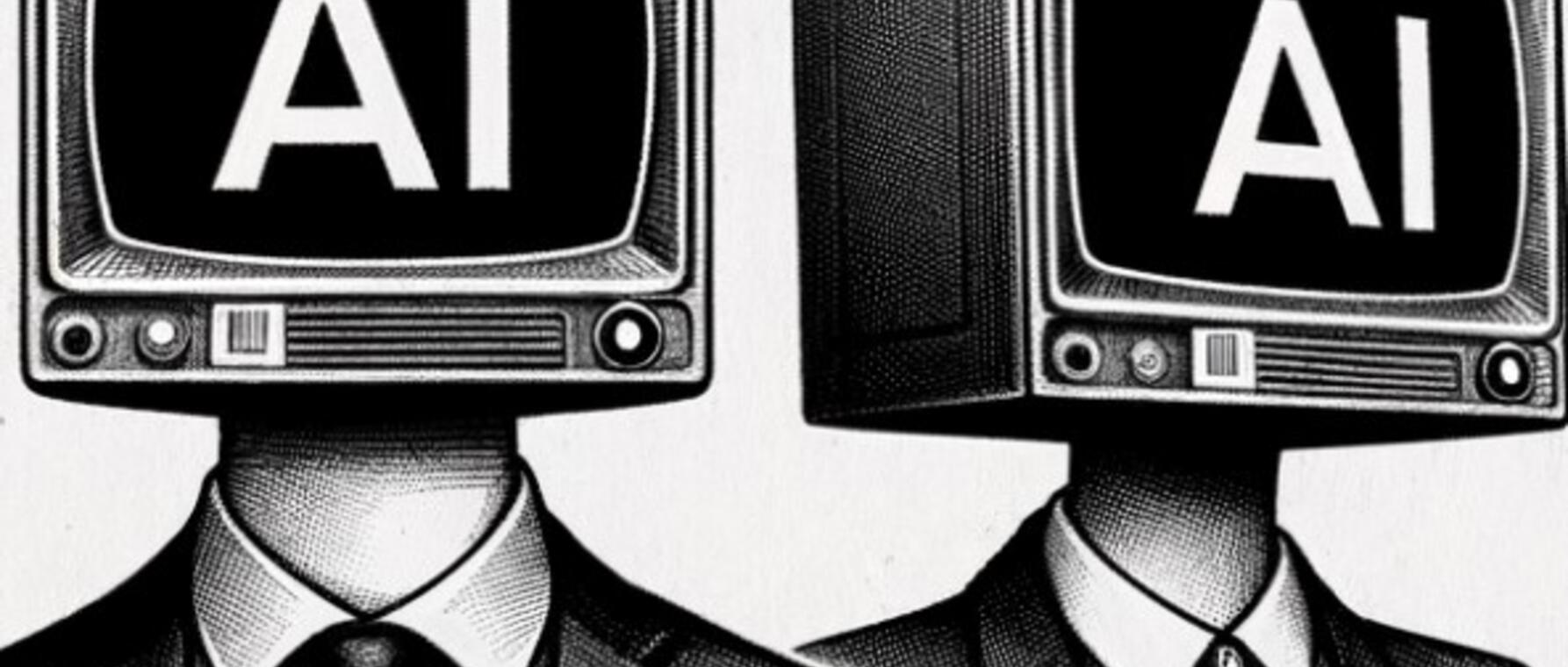
Research at Risk: Since World War II, universities have worked with the federal government to create an innovation ecosystem that has yielded life-changing progress. Now much of that work may be halted as funding is withdrawn. Find out more about the threats to medical, engineering, and scientific research, as well as how Harvard is fighting to preserve this work—and the University's core values.
Technological disruption of human occupations is nothing new. In recent decades, blue-collar occupations have borne the brunt of the upheavals—think of all the factory workers now working at Wal-Mart thanks to the integration of robots on assembly lines. But all that may be changing now. Given artificial intelligence’s ability to do thought work—from crafting feature stories in seconds to writing and editing computer code—disruptive innovation is now coming to a college-educated profession near you—and me.
Feeling concerned? Take heart. Harvard's Isabelle and Scott Black Professor of Political Economy David Deming says AI is here to make us more productive, not take our jobs—at least not yet. The co-author of the recent paper, "Technological Disruption in the US Labor Market," Deming says that thanks to technology, every small businessperson or professional can now have an indefatigable digital assistant, one with a flawless memory, encyclopedic knowledge, and lightning-fast response time—and one who will never ask for a raise or even a wage.
Deming, who received his PhD from the Harvard Kenneth C. Griffin Graduate School of Arts and Sciences in 2010, spoke recently about artificial intelligence and its impact on the labor market during an event for the School’s alumni at the Harvard Club of San Francisco. He was interviewed by Harvard Griffin GSAS Dean Emma Dench, whose questions were often submitted by audience members.
So, let's start very basic. Can you just tell us what is a GPT? And I know what it stands for, a general-purpose technology. What is it? And give us some examples from the past.
Sure. Well, thanks, everybody, for coming. It's great to be here with you. I’m really happy to be here talking about AI. Let me answer your first question, Emma. So actually, GPT has two meanings. The company OpenAI released the model called ChatGPT, which many, many of us know. In that case, GPT stands for generative pre-trained transformer, which is the model itself. That's a statement about, it was very intentionally a play on the other meaning of GPT, which is general purpose technology.
And that's something defining; what is a GPT is an art rather than a science. But the general idea is that economists, historians, economic historians who have studied the history of technology and how it affects work and society have identified a category of technologies that have had outsized impacts on us. And they tend to have a set of features that collectively conclude us, lead us to conclude that they are general purpose technologies.
And so there are things like new power sources, steam power, electricity; the personal computer is another example. These are things that are general in the sense that they are actually, you want to think about them as base layers that enable, for example, other complementary technologies. So when you have a new source of energy, for example, the electrification of US households enabled the invention of the microwave, the dishwasher, the refrigerator, the vacuum cleaner, a bunch of innovations that saved people a ton of time.
It's kind of prosaic now to think about the dishwasher and laundry, but actually, if you think about the time savings and the way that enabled, for example, two members of the household to work, or technologies like indoor plumbing that enabled us to have clean water, reduce the incidence of disease. These technologies were really at the root of economic growth in developing and developed countries at the beginning of the 20th century. And they had their roots in these general-purpose technologies.
And so the question is, is AI a GPT? And there's no certain answer to that question. But one way to think about it is, does it have the kind of widespread application that, let's say, electricity does? Do we see it being used in a variety of different jobs, in a variety of different ways, in the same job? Does it have that ability to be the base layer on which many complementary innovations are developed?
I think there's some early evidence that the answer might be yes. And some of it comes from studies, kind of one by one saying if we give people on the job access to ChatGPT, Google Gemini, some generative AI model, how does it improve their productivity? And this ranges from helping you write to helping you write software code, to helping you provide customer service.
And the answer seems to be it improves productivity for those people by around 25percent, which is not a huge number, but it's also an early-stage technology. And it also seems to be widely applicable. My colleagues and I a few months ago conducted the first nationally representative survey of generative AI usage among workers, people at work and at home and found shockingly high rates of usage. Something like a quarter of all people on the job reported they used generative AI at least once in the last week on the job. And the use of it is quite widespread.
So it's most common in software jobs, not surprising, management jobs, kinds of business. But almost 20 percent of blue collar workers reported using AI at least once in a while. It's very common. At least one in eight workers in all types of occupations are using it, so that's the kind of thing that looks general purpose. The question we don't know yet: is it going to be 25 percent productivity, which is great, but not the kind of thing that transforms the economy. Or is it going to be 200 percent to 300 percent productivity, like for example, what happened when we mechanized agriculture? So the move from using draft animals to using tractors led to a complete revolution in farming. We don't quite see that kind of productivity growth yet, but the technology is only two years old.
So, when you talk about the increased productivity, are you seeing AI as more disruptive to the labor market than the sorts of technologies that you were talking about?
A hundred years ago, about 40 percent, two in every five jobs in the US, were in agriculture. Now it's less than 2 percent. In the mid-20th century, about 40 percent of installation, what people would call blue collar, so either manufacturing jobs or construction, installation, maintenance, repair, labor. Now it's 20 percent, so a halving of those jobs.
And so you've seen over time really seismic changes in the US labor market. The middle of the 20th century was a time of tremendous disruption. We went from people working on the railroads. My grandfather worked on the railroads, and nobody works on the railroads anymore. We transitioned to the personal automobile. And so you kind of had railroads converting mostly to cargo and people. It's like a whole category of transportation jobs disrupted, changed.
And so this kind of change is the norm throughout history. And so the bar is very high. So, AI could be quite disruptive by our common standards, but it wouldn't necessarily rank highly in historical perspective. It's going to have to do a lot. It may get there, but it's going to take a while for us to know whether it's actually going to be on that scale.
Interesting.
I should say one more thing about farming. Yes, it went from 40 percent to 2 percent, but it didn't happen overnight. It was a percentage point decline of 2 percent per year for 20 years, 40 years or whatever. It's just a very gradual. If you look at the line, it's just a very gradual disruption. And so that's kind of the norm even for the most disruptive technologies.
So we've been through a lot in recent years. And maybe it's only just beginning. But we just faced the massive disruption of COVID-19. And so how do you distinguish between the disruptions and the recovery associated with COVID-19 and the disruption associated with AI as a new technology? How did you tease that apart?
Very carefully. Yeah, it's a great question, because some of the—so I've recently written a paper with Chris Ong and Larry Summers called "Technological Disruption in the Labor Market," which takes a long-run perspective on this question, some of the facts I just mentioned come from that paper. And one of the things we noticed is that actually, if you look at the 2000s and the 2010s, this is a period where people were saying, oh, the labor market is just changing so rapidly, rapidly than ever before. It actually wasn't by any objective sense.
So we calculated a measure we call churn, occupational churn, which is: how different is the structure of the labor market in one year compared to the next, like, do you see a lot of disruption in the nature of jobs? And by historical standards, the 2000s were the most calm period in the last century, actually. And yet, if you look at the last five years, so you just look at our measure of churn since 2019, it's actually back up to levels not seen since the 1970s.
And so the question is: is this a COVID blip or is this kind of a new normal? And obviously we don't know for sure yet, but I think when I look at the data, I think it's more likely the latter than the former, actually. So I think actually it might be here to stay, even though as an economist, I think I'm supposed to be skeptical. We've seen, but there is some chance it is for a couple of reasons.
So one is we've seen big changes in the labor market as a result of investment in AI already. So people date these things differently. But somewhere around the mid 2010s, large companies started getting serious about infrastructure investment data centers, and then what I would call predictive AI, so not using generative AI, but the idea of, for example, using computer vision to predict, to optimize the movement of robots around a factory. So like Amazon bought Kiva Robotics, basically optimized inventory management using algorithms to figure out how to get the right products to you at the right time and things like that.
And that was a really disruptive change. And you can see it in the data. So, we've seen an increase in private R&D spending as a share of GDP of like 35 percent, 40 percent over the last 5 to 10 years, and an increase in just investment in information processing and software. And also the share of all jobs in the US economy that are STEM jobs, so science, technology, engineering, and math went from about 6.5 percent in 2013 to more than 10 percent today. So that's a 50 percent increase in the share of all jobs in STEM.
So that's not even thinking about the labor market impacts of using AI. That's just the fact that these huge companies are spending billions and billions of dollars investing in AI, and they're hiring tons of technical talent, and you can actually just see it in the data directly. So that's one reason why job churn is up. The other reason is that if you think about low-skilled service jobs, two major categories, I would say low-skilled jobs that don't require a lot of education or specialized training.
So two major categories are food service, so either waiters and waitresses or fast-food counter workers like food service and preparation and then retail sales. So these are two categories that behaved very similarly in the 2010s. They're about 7 percent of all jobs, something like that. In the pandemic, they fell off a cliff for reasons related to health. But actually, jobs in food services dropped more steeply than retail sales jobs, 35 percent, 40 percent decline in employment in those jobs, like in 2019-20.
If you look at those two lines, not, they're just totally for cover. They haven't totally recovered to their previous height, but they're back on the upward trend. Retail jobs are not. They are just totally flat. They have not recovered at all. And I think the reason for that is that people got, you know, online shopping was around for a long time. It's been around for a long time. And yet people have habits, and maybe you're used to going to your local retail store and you just get used to it. And you don't want to shop online because what you're doing now is fine.
But then in the pandemic, you were forced to do it. And then you realize, hey, actually, this isn't so bad. And Amazon made huge investments in same-day delivery because they knew this was happening. And so you got used to buying things online. And so stores said, okay, actually we're not going to reopen. Or if we reopen, we're going to hire fewer workers than we did before, because maybe you come to the store and then you buy it on your phone. People do that. And so I think there's a sense in which the pandemic has actually directly accelerated the pace of change in the labor market, because it kind of mixed up people's habits.
So I want to move, inspired by what you were just saying about food service workers and retail workers, to talk a little bit about the unevenness of take up, of use and application of AI and about inequality. And maybe start with a broader question about what the demographics look like, and how this relates to inequality in the workplace.
So as I mentioned earlier, we conducted a survey of generative AI usage. And one of the things we were interested in are the demographic patterns of who's using it, who's using it more intensively.
So we asked a lot of questions about the frequency of use, and at work versus at home and things like that. And what we found was a pattern that actually is very similar to other patterns of technology adoption. If you look at things like who used personal computers first or who used the internet first.
More educated people adopt technology at faster rates, and we see that with generative AI. So if you have a college degree, you're about twice as likely to be using generative AI as if you don't have a college degree. Men use it at higher rates than women. So men are early adopters of technology. That was actually a little bit less true with the PC, but that's because a lot of secretaries and administrative assistants, that's a heavily female occupation, they were some of the earliest adopters of PCs, so actually went in the opposite direction in that case.
So more educated people and then people in professional occupations, so think business management, STEM, versus retail, food service, et cetera. So that's the general pattern of AI adoption. We do see some evidence on college majors, so people who major, it's kind of what you would expect, people who major in STEM or in business are more likely to use it than people who major in humanities, for example. You can be an exception to that.
I won't take it personally.
I'm just—I just report the data. So as it relates to inequality, I don't think it's obvious at all, actually, because even though we see these patterns, you might think, oh, well, the more educated, better-off people are going to use this technology more. That might be true, but it doesn't follow that they'll benefit more from using it. Actually, a lot of the evidence we've seen from studies of organizational or personal use of AI is that it—as I mentioned, it increases productivity by about 25 percent on average. But that increase is uneven. It actually tends to give a bigger boost to the lower performers.
So it's much better at getting you from novice to average than it is to getting you from average to great. And you see that in a lot of areas. And so that actually is a hopeful story for inequality, because AI could be a leveler. It's basically going to allow you to—I wouldn't say be an expert, because you're kind of used to mimic expertise.
So some people have used the analogy of thinking of AI like an exoskeleton. I don't know if any of you guys are like comic book fans or anything, but the idea is it's like an augmentation suit. When you're using AI, you put it on, it lets you do things that you couldn't do otherwise. But when you take it off, you can't do those things anymore. But it basically enables you to achieve a higher level of expertise in less time than you otherwise would. And that obviously benefits non-experts more. So that's a more hopeful story.
Will AI allow most people to work much less or will work expand?
I think the answer depends on what you mean by, “will it allow you to work less?” So, I think the answer is almost surely yes. Actually, every major technology has allowed us to work less. If you think about what share of human labor is actually required for survival, it's almost none.
Like most of us in this room have jobs that are not necessary for the survival of the human race. We're producing like, even I'm producing like in some sense, we're luxury goods. Many of us are. And so do you need it to—are you going to need to do that much work? Like, how much work do you need to do to feed your family? It's a vanishingly small share of the actual work you do, but people still work. Why? AI will enable us to buy things, and you want to have social status. So what I suspect will happen is that AI will enable people to produce more in less time, but that many people will still want to run the rat race, because many things we do are about, and just maybe a more generous view is like work is not only about survival, it's about fulfillment. It's about meaning. It's trying to create something. And so I think many more jobs are actually like that than they used to be.
So an example: I was on a panel, so we had Junior Family Weekend at Harvard College a few weeks ago, and I was on a panel talking about generative AI with some parents. And there were some questions about is AI going to take all of our jobs kind of thing? And I said, well, these companies, their explicit purpose is to build artificial general intelligence. So let's say they succeed. I still don't think it'll take all of our jobs, because intelligence isn't the only thing that people care about in work.
And there's a lot of jobs where it's not even the main thing you care about. The example I gave is, I coach my daughter's soccer team on the side. OK, I don't know anything about soccer. I really don't know anything about it. It's like they call me Ted Lasso. They joke with me. And, I could probably enlist an AI assistant to help me with substitution patterns, strategy, whatever.
Maybe I could even have the AI coach for me. Nobody wants that because they're not looking for me to be the best coach. They want me to build a relationship with their child. They want to have someone that can talk to and like, so the value of that job, you're looking for, jobs like that, coaching, mentoring. The point is not intelligence. The point is to make a connection. That's what you're looking for. And so you would never want an AI coach, even if you could have one. So I think that's the way to think about it, is that for jobs where intelligence is the primary thing, maybe they'll come in. But not every job is like that.
There's a very, very interesting question that's come in about that invites you to reflect, thinking about your daughter's soccer team a little bit, but invites you to think about how generational factors relate to this huge technological change. Can you teach old, old dogs new tricks? And also just the question of whether, I guess, putting it in my own words, whether society has to age into the technologies as we're all raising digital natives as such. I'd love some reflection on that.
That's a great question, near and dear to my own heart, I am kind of a like, wannabe techno geek. Not that I know everything about it, but I want to experience it. So like my first item on my bucket list when I came to San Francisco was, I want to ride in a Waymo. Like, that was like the first thing I wanted to do.
And when ChatGPT came out, like I wanted to use it. Not that I want to—I do use it somewhat regularly in my job, but I wouldn't say it's essential to the job. I use it more to explore and experiment, to try to understand what's like it. So I would encourage all of you who feel like you're an old dog. I feel like I'm an old dog who needs to be taught new tricks. You can do it. It's like it doesn't need to be mission-critical.
But there's a—to me, at least, there's a joy and a wonder to seeing what the future is likely to be, even if it's going to take, as I said earlier, longer to come than most people think. I still want to know where it's going. And so I would say, that's the thing that younger people have that we don't have, is that they still have some sense of openness about, maybe I could do things differently. And we can actually recapture that. So I would encourage—I would encourage you to try.
Having said all that, I do think the history of technological change is that it typically is about aging in and aging out of cohorts, rather than people suddenly adopting, to be realistic about it. One of the reasons why I decided to conduct this survey of generative AI usage was when the technology first came out, I would talk to my colleagues about it, and most of my middle-aged professor colleagues know about AI. Most of them have even used it, but they view it mostly as a curiosity, like, oh, you know, it's not that really useful. And like, I played with it a little bit and whatever.
And then I would go and I would ask the students, again, because I live on campus, I live at Kirkland House. And so I'd walk into the dining hall and I would ask people, are you using AI? And I quickly learned you don't ask if they're using it, because they're sheepish about it. I said, how many of your friends are using it? Or what share of your friends are using it? Ninety percent, 100 percent, everyone's using it. Everybody, okay?
And so I thought, that's interesting. Something's happening here. And so that was the inspiration to do the survey. I think that has really important, I mean, I think as what's implied by the question, whoever asked it, it's a terrific question like, this is happening. And it's happening through the younger generation, and we can't pretend it's not.
I feel some anxiety about that within the College, because I don't think we really understand how to respond to it. I don't think it's fair to have expected us to have a strategy already because it's so new, but I think we need to figure it out. But probably the history of this is we won't really do a great job in, like, the digital natives who come along will replace us and they'll figure out how to do it better. That's probably what's going to happen, even though I hope it isn't how it actually happens in this case.
[Let’s say] another pandemic comes rolling in. Do you think there's a prospect for AI to help lessen the economic disruption of that future pandemic compared with the last one? Can it really help us with that?
No, I don't, because I don't think that the failures of the pandemic were technological. I think they were political and social. And so I don't know that it would make it worse, but I didn't come away from the experience of the pandemic feeling like technology was, would have been, would have made it better. I think it was about social trust and effectiveness of government, which is really just people working together, trying to solve a problem.
I think a lot of great things happen. I guess the only thing I can say is I think there's a huge positive thing I can say on this is that there's a huge—probably it could end up being that the most important application of AI, of generative AI in the end, is medical innovation and maybe even materials innovation. So, there's a wonderful paper by a graduate student at MIT, Aidan Toner Rogers is his name, where he studies a company that's basically inventing new material compounds.
And they have this AI program. It's not even like an LLM, it's like an AI, but it's a—well, it's a generative AI program. It's not like ChatGPT or something. And it basically tries to predict what might be some good material, and it leads to huge compounds out of it. So it's kind of quasi-automating the scientific discovery process. And it leads to huge increases in the productivity of scientific discoveries. It's basically giving researchers promising leads.
And then the researchers, so the thing is it makes them a lot more productive, but it makes the researchers a little bit more unhappy because it actually takes the creative aspect of the job away, which is coming up with the idea. And instead, what the researchers have to do is use their vast corpus of knowledge to say, well, which of these ideas AI generated are actually good leads? And then do the kind of grunt work of figuring that out. But what it shows is that you could imagine a combinatorial explosion of innovation in medicine, and anything where you're basically just trying to combine materials.
And that's a lot of the history of discovery in science is like somebody experiences serendipity and discovers something amazing. Like, I don't know if you know this, but the GLP drugs like were basically invented through Gila monster venom. Did anybody know this? There was a great article in the New York Times about it last week. So it turns out that Gila monsters are these desert animals in New Mexico, and they have this interesting property in their saliva, which is that they have venom in it. And when they bite an animal, it kills the animal, but also when they consume it, they don't need to eat for like three months. And the chemical compound in their venom is, actually was, actually the basis of the innovation of the drug that has become Ozempic.
And so that's a totally—and there are these people who are working on it for years who like discovered and were put it on the shelf and then somebody else. And so scientific discovery is so haphazard. But what if we could use AI to supercharge it? So that to me, that's a hopeful thing. It's not a pandemic thing. It's like, what if we could prevent the next pandemic because we could use AI to automate the development of a new vaccine?
Artificial general intelligence: can you talk a bit about what that is? And there are various predictions for when that might happen?
So artificial general intelligence is the idea that is the explicit goal of companies like OpenAI and Anthropic and some others. They're trying to build giant models that can exceed, meet or exceed human performance on a variety of tasks. The idea is you reach AGI when you can basically be smarter than people in a general way. I feel that depending on what you want to call AGI, we've either already achieved it or we will never achieve it because the definition of intelligence is not that clear.
If you think about it as an IQ test, well, AGI is already with us. Like you can give—even like certainly the frontier models like GPT4 or Claude, like you can give it the LSAT, the SAT, the bar exam, like any test that people think is associated with being smart and it will ace it. So in that sense, we already have AGI, but that's not really what the tests are.
To me, they're not intelligence. They're like a way for people, people who score higher on the test tend to be more intelligent. That doesn't mean the test captures everything about intelligence. Intelligence to me is about is about learning efficiency. That's the way I would think about intelligence. So, how good am I at figuring out something I've never seen before? That's, to me, a good working definition of intelligence.
And by that standard, they're not particularly close because the ratio of the amount of data you need to get the insight is way off for machine any of these models compared to educated guessers. Give me just a little bit of training data, I can figure out what to do. And that means I'm more intelligent than the model. So that's my definition. That's a different definition. Steal our money from our AGI, there's this worry that the machines will get so smart, they'll outsmart us. And they'll steal our money from our bank accounts. And there's all these kind of doom scenarios that could be plausible.
And if the probability of that happening is only 1 percent, well, we probably ought to buy some insurance against it, because that's like the end of the human race, and that's pretty bad. So I think we should take the tail risk seriously, but I have a hard time thinking about it concretely.
Currently it costs so much to address marketing, sales, legal issues, operations. How might they really, really benefit from using AI?
Here's the way I think about using the models for what it's worth. This is how I use them. Imagine you had a research assistant that had an encyclopedic memory and was very intelligent, but had somewhat suspect judgment, by which I mean, kind of knowing when you know and when you don't know, knowing context.
But it never gets tired. And you ask—have what you want, and you can do it over and over again. And it's super quick, that's what it is. It's having a conversation, you should be having a conversation with the model because it's like a person. It's not like a computer program. It's like a person that has an odd set of characteristics, but that means you can benefit from it.
Now how that helps small businesses, I think it's possible to—let me say it this way: If you're just using the model to do one thing once, it's not that big of a difference versus another person. But the thing is, it never wears out. And like you can do it. So anything you need to do more than once, you should just write down—just ask the model to do it for you and build a little agent that does it for you.
So you can build a customer service agent. You can build an agent that will take data and put it into a spreadsheet. And then if it doesn't do it right, you can just tell it, you did it wrong, do it this way instead, and it'll figure it out. So that's kind of like if you had a research assistant that was doing it, except that there's no communication problems. It understands you. So it's like a really efficient way to have a staff.
Larry Summers was saying, I think it's right is that like when he was Treasury Secretary, everybody understood that when he wrote a letter to the president recommending policies, it wasn't literally him writing the letter. He was overseeing the writing of the letter, but he had a whole team to look up the research, to fact check everything, to make sure he's like, now everyone has a team, everyone has the Treasury Secretary. And I think that's the right way to think about it. You've got a team.
And so if you're a small business, you can scale up by using the fact that you have a cheap team that you don't have to pay, you only have to pay $20 a month. And it's not going to get mad at you or ask for a raise or unionize. So you should use that to increase your productivity as an organization.
So here's the downside of that. How might any of us AI proof our job, what you just said.
I might regret saying this, but I'm really not that worried about any of us getting replaced by AI in the short run. I mean, who knows, 20 years from now. But I actually think the technology as it's currently used is much more of an augmenter than a replacer, because it's not like, first of all, anything that exists in the physical world cannot be replaced by AI right now. Maybe someday, but it's not close, really.
Like, if all you do all day is write emails and write documents, like maybe you could be replaced. But like most people don't really do that. You communicate about it, you sell certain things, you persuade people, build relationships like you do a lot of things that AI can't do. But what it can do is help you do your job better, so you can focus on the things that only you can do.
And there's a cliché people say, although I think it's a cliché, but I think it's accurate, which is you're not going to get replaced by the AI, you'll get replaced by a person who knows how to use the AI better than you. So that, I think, is the better way to think about it, is that what's going to happen is in white-collar jobs where AI is useful, expectations will ratchet upward. So, your boss is going to start expecting you to be more productive because they know you have access to this tool.
You've still got some time, but eventually, like everyone's going to be using it. I mean, I think a lot of people are using it. They're just using it on the sly. And maybe now that your coworker isn't using it, you can save a bunch of time and then go to the bar earlier and get your work done faster. That won't last long. What will happen is the company will say yeah, I'm expecting you to use this. And like I don't need three days to write this memo, you need three hours and expectations will ratchet upward. So that's I think the risk, not replacement, not yet anyway.
Are there any very cool applications of AI to specific businesses that you want to share? One of our guests has particular questions about the construction industry, which you may or may not have thoughts on but any industry in which either currently there are some untapped possibilities. You can feel free I think—this is one of those to fantasize about.
I’ve been interested for a long time—Linda mentioned in introduction the Skills Lab. I've been building these measures of soft skills, how do you know if someone's good in a team, for example. I've been interested in teamwork for a long time.
And my team and I developed this method basically to identify individual contributions to group performance. The idea is if Linda is on a team with two other people or three other people, and the team does well, was Linda responsible? Was somebody else responsible? Was it like team chemistry? Like who actually is the person that's contributing and who's not? And that's a hard problem to solve.
So what you have to do is you have to put basically put people in a bunch of different teams and see if they always make the team better after accounting for the skills of the other team members. So, we figured out how to do that. It works really well. It turns out there are people who are really good team players, we call them.
And it turns out that it's very well predicted by your score on something called the “reading the mind in the eyes” test, which is a widely used measure of emotion recognition. So basically, can you read other people? It turns out to predict whether you're a good team player, which is that's a cool result in and of itself. Nothing to do with AI.
But the problem with doing that is so we've been trying to build these measures that other people can use, and it's really cumbersome to put people in teams repeatedly, like with artificial intelligence, where it takes hours and whatever. So we thought, well, could we actually simulate this with artificial intelligence agents? So what we did was we did the team thing and we established some ground truth. We have a sample of people we know who the good team players are.
And then we give them like three AI agents to work with. And we ask the question, are the people who are good on human teams also good on AI teams? And the answer, astonishingly, is definitely yes. Like the correlation is almost one. It's almost perfect. And basically the reason is because in the task we have people do, what you have to do is you have to surface information from people. So everybody in the team has some information that's needed to solve some problem, but not all the information.
So you have to talk to each other and figure out who knows what. There's some extraneous information. So it's like a conversational thing. And people who are good at eliciting information from other people are good at eliciting information from LLM agents. And so that's kind of cool. But then I got interested in this question of, well, that's just like a generic agent pretending to be a person. What if you could actually train an agent to be a specific person, not in the sense that you want to trick someone, like, not like I'm going to fool you and think I'm David.
But suppose that your boss is Linda. Sorry to pick on you, Linda. Linda is your boss, and you want to simulate having a difficult conversation with your boss. Let me give you “Linda bot.” And the bot acts like Linda, or maybe it just acts like a generic boss or something. And you can use the agent to simulate the kind of conversation you would like to have that is actually a high-stakes conversation.
And so I think actually these agents are really good for that kind of thing. I think simulation is going to be huge now that we have AI. Why? Because if you think about where it already exists, it exists in the industries where failure is extremely costly. So flight simulators, surgery, you simulate surgeries because the cost of a mistake is very high. And so you build these really expensive simulations because the benefits outweigh the costs.
But now, if the cost of the simulation has gone way down, everybody should be doing it. It's a better way to learn. So I think we're going to be using AI agents to create much more immersive learning environments, possibly within firms learning how to have conversations with people, how to work together. And I'm excited about that.
One of one of our guests was wondering about teaching soft skills through role play, and that's exactly what you're talking about. I really like that. And there is a whole flurry, you've segued beautifully onto a whole flurry of questions from earlier this evening and escalating right now about education and also what AI does to our minds. So maybe just starting with the basics. How does this several questions about, again, as we were thinking about the younger generations, even back to high school, how does high school...? Maybe it's even before that, and college, what changes do you do you think are needed?
Yeah, so this is a topic near and dear to my heart. I have a kid in high school right now, and I can tell you what they're doing in her school, which is the teachers have decided that the way they're going to prevent people from using AI is that when you do your assignment, you have to write an essay in her English class, you have to write it all in a single Google Doc. You can't copy and paste.
So I mean, that's not a good solution, because all you have to do is have one window open where you ask the AI a question, you just type the thing that you see and you can get around it. So it's not a very sophisticated response. I understand why they're doing it, because they want to—I mean, cheating has been around forever. But what this does is it lowers the cost of cheating to 0, because you can just ask the AI a question and it'll give you something.
And you can just—I think the temptation is so great, that I'm pretty worried about it. I think we should be worried about it. I think it's not good for people to use AI to make learning easier. That's what I always tell my students and my kids. If you're using it to get around the hard work of learning something, that's a bad cure. To deepen your understanding, if it's making you think harder, that's a good use. That's your signpost for whether you're using it appropriately. If it's making your job easier, it's bad.
And so I think we have to, first of all, acknowledge that this is happening already, everywhere. And second, we have to as educators, we have to probably do some things we should have been doing already, and some of us are, which is to design the kinds of classroom experiences and assessments that are AI-proof in a narrow sense, but are also ask more of people than just regurgitating knowledge. But also even synthesizing I think is not enough. We need to ask people to apply knowledge to different contexts.
So for example, I used to—I used to teach a big class about economic inequality. And I would ask people to write these essays that would—I'd ask them a question like, can we reduce economic inequality in the US back to 1970 levels, if we increase the top marginal tax rate to what it was in 1970? What do you think? And so they would write an essay about what the evidence shows. And like, I would pat myself on the back about the cleverness of the question, but AI could do that easily.
So now what we need to do is say, OK, you need to know that, but I'm going to ask you to come to class and give a presentation about it. I'm going to ask you to convince your colleagues. Set up a debate in class and have two people argue either side of it, and then the class votes on who's right or something like that. And that's where you actually have to demonstrate mastery of the material in an interactive setting. So it's both AI-proof, and it looks much more like what you have to do in the real world.
Because the other way you could go is you could just have a blue book essay that's like old school, like, but that's to me, that's going backwards. That's saying we're not going to use any technology because we want to make sure that you're not cheating. But it's also kind of like dumbing down the goals of education. So I think we just need to push harder on, like encouraging deeper engagement with the material in a way that's also AI-proof. And I mean, I'm pretty worried—I'm pretty worried about what's happening all across the country right now, not just at Harvard, but everywhere. I think there's a lot of shortcuts happening in education.
A guest wants you to think about the impact that AI might have just on our brains. So will it make us. I mean, I'm glossing? Will it make us stupider? Will it ruin our memory? Will it have an effect on critical thinking? And the analogy which I think is beautiful that our guest gives us is that it wasn't that long ago when we all had whole sort of repositories of seven-digit phone numbers in our heads, and that's gone. And I know in my field there's a lot of reminiscing about oral poetry or when we all learned poetry, et cetera. So any reflections on any early indications of what this technology is doing to is doing to our cognitive abilities? And is that different from other recent technologies?
You can see this happening in the past at other times. Like, one thing I like to do, maybe this tells you something about me. I like to look at old curricula, either college curricula or first grade, like see what they were asking people to do at a similar timing 100 years ago.
And if you look at entrance exams for Harvard or something for 100 years ago, there's quite a lot of questions that I myself couldn't answer, but they tend to be very fact-focused and like a lot more memorization-focused and less analysis and synthesis. So there's a lot of reminiscing about a time when education was a lot of rote memorization. It was hard, but that doesn't mean it was useful necessarily.
So I think it doesn't need to be the case, like AI will change. In the same way that we don't need to memorize phone numbers anymore, we may not need to know grammar or spelling. And you already see this, like, my kids are much worse spellers than I was because they have spell check. That's obvious. But that doesn't mean they're not thinking. I think the question is, what substitutes in? And so I continue to think that our metric ought to be, are you really engaging with material? Are you learning? We need to be able to measure that better. Sure.
But I think a good statistic for is, do you have it--do you have your students engaged? I think if people are engaged in learning, even if they're learning in a different way or learning different things, it's probably going to be okay. Maybe they're learning something that's even more useful for today's world. But what I don't like to see is people going, you give them homework and they go home and they like type it into a ChatGPT window. They turn it in, they're not engaged. They're just using it to substitute for their own effort.
So I guess I think the challenge as educators for us is to make learning engaging, to basically compete away the temptation. It's on us to say, hey, look, you could use this to take a shortcut, but actually it's going to be much more interesting if you don't—it's enjoyable and entertaining. Like, we might not want to think about ourselves as entertainers, but good education is enjoyable and entertaining and engaging. And so I think we just need to step up our game.
I love that. And that brings me to another question about creativity and creative work, and thinking of all the issues on with the Writers Guild in recent years. And I wonder if you have any reflections on both the vulnerabilities and what the possibilities may be as well.
Yeah, so it's a great question, because I think it really depends on—you could think about Hollywood or any businesses having some components that are what I would call front office and back office. So I don't think AI is going to replace the actors in Hollywood because people get attached to those people. Like don't want AI Ben Affleck, you want Ben Affleck or whatever, whoever the star is, Tom Cruise or whoever it is. Maybe I'm dating myself by picking those names.
So you can already do that. Like you could have AGI actors, but people don't really want, they want to see the people they know. And so I think the issue with the Hollywood writers is they're mostly behind, people mostly don't know who they are. And so that makes you more at risk for being replaced because there's no reputational benefit. Basically there's no, oh, I want to see a movie by this writer.
Sometimes there is, but I think you'll see this in media too. People will push back against being replaced by AI is by establishing reputation. And I think you'll see this in media too. It'll be like, when I can write—like, I have a Substack. And I could use AI to write it, but I'm very invested in having my own, like I like actually, I actually like doing it. And there are big costs. There would be big—I mean, I'm not that big of a deal, but if somebody writes something who has a big audience and it's revealed that they're using AI to write it, that's a big reputational risk for them.
And so people are going to be staking their reputations on like, I'm not—either I'm using it in this particular way or I'm not. And here's the authentic me. And I have a voice and you can count on me. And it's like you're having a conversation with me when I write something or I'm on a podcast or whatever it is, I think you're going to see that become even more important, because that's AI-proof. Like, I don't want AI Deming, I want Deming.
And so in order for that to work for me or for anybody have to have a distinctive voice. You have to have something that is distinguishable from, like the average of the internet, which is kind of what ChatGPT is in a sense. So I think you'll see more, you have more voice in all aspects of creative culture. You see it already, but I think you're going to see it even more. I think that's going to be the most important thing.
Here's another fascinating thought about an application of AI which might be fraught with difficulties. Can you ever imagine it being used in court and as a basis for forensic decisions, et cetera?
Well, I mean, in the same way that DNA took over, I would expect that if AI is better at figuring out the truth of the matter, then it ought to be used. I mean, I think to me, that's a case where it could clearly do some good if it's accurate. There actually is a lot of usage of AI, a different kind of AI, not generative AI, but algorithms that predict things.
So there's been a big movement and then retrenchment of algorithms that will essentially tell you things like how likely is this person to commit a crime if I release them on bail. And people have used these algorithms to make—judges use these algorithms to guide their decision making about whether to release somebody when they're awaiting trial. And there's been a lot of pushback about racial bias in these algorithms and things like that. So that's a very active area, both of scholarship and of practice. So I think AI can and should be used very carefully in those situations.
Final question in this more formal setting before we move on to drinks and more discussion. So I'm a dean. Imagine I have an unlimited budget, if only. If I give you David Deming, an unlimited budget at Harvard, and you have to do something around, it's an AI project, what would you do?
Yeah, unlimited budget is almost hard to think about. So here's one crazy, maybe foolish idea I have for how to step up our game at Harvard with AI. So I think that we should be holding professors' feet to the fire to ensure that the way we teach is kind of like, I wouldn't even say AI-proof. I would say is AI-compatible in the way we grade.
And so one thing you could do to ensure that happens is you could—let's say you could—we don't want to mess with tenured professors, but you could say this is voluntary. You say, I'm going to let you opt into a program that we are going to—we are going to secretly submit AI-generated answers to your classroom assessments, and we're not going to tell you when they are. And we're going to either see if you can spot them, or we're going to give you feedback about whether they are graded similarly.
And we need to—and we're not going to call you out in some public way, but we're going to tell you, look, here are the AI assignments. Were you able to recognize them? Did you actually—and if not, is your classroom really AI-compatible? What can you do? And I would make that, things like that, it's kind of like a friendly nudge. Maybe that's a stupid idea. That would never work.
But the idea is to really nudge us hard into the idea that we need to take this seriously. And I do think there's a way in which well, let me say the hopeful version of it, Emma: I think that there is a way that we could use this moment to revolutionize in a positive way, the way we educate, that you could actually use the tool to deliver a better, more engaging classroom experience.
And this seems like a good opportunity because sometimes the worst decision is no decision. And that's what we're doing right now is no decision. And that seems to be a kind of a disaster path to me. So why not use the opportunity to really have some fun and, like, design a better educational experience?
The Colloquy podcast is a conversation with scholars and thinkers from Harvard's PhD community on some of the most pressing challenges of our time—from global health to climate change, growth and development, the future of AI, and many others.
About the Show
Produced by GSAS Communications in collaboration with Harvard's Media Production Center, the Colloquy podcast continues and adds to the conversations found in Colloquy magazine. New episodes drop each month during the fall and spring terms.
Talk to Us
Have a comment or suggestion for a future episode of Colloquy? Drop us a line at gsaspod@fas.harvard.edu. And if you enjoy the program, please be sure to rate it on your preferred podcast platform so that others may find it as well.
Get the Latest Updates
Join Our Newsletter
Subscribe to Colloquy Podcast
Simplecast